A research team in Germany said that an artificial intelligence (AI) model based on routine data of F-18 FDG-PET/CT scanning can improve the accuracy of staging treatment for patients with metastatic lung cancer.
A research team led by Dr Julian Rogasch of Charette Medical University in Berlin has developed a machine learning tool to help evaluate mediastinal lymph node metastasis in patients with non-small cell lung cancer (NSCLC). They found that the model was more accurate than the visual score of PET, and suggested that it could help doctors make more accurate treatment plans. Their results were published in the February 23 issue of the European Journal of Nuclear Medicine and Molecular Imaging, and they wrote that the model can be used as a network application to further promote verification.
Dr. Rogasch and his colleagues wrote: "This model was developed for routine clinical care, and the probability of N2/3 disease (that is, whether there is N1, N2 or N3, which is the key to determine stage II and III diseases) was estimated according to the results of F-18 FDG-PET/CT. This is based on routinely available variables, most of which are already part of the F-18 FDG-PET/CT report in routine clinical nursing. "
In patients with non-small cell lung cancer, accurate imaging of thoracic lymph node metastasis is very important for treatment planning. The authors explain that patients with N0/1 disease usually need surgery, while patients with more extensive N2/3 disease need multimodal treatment. They pointed out that although F-18 FDG-PET/CT is the most effective method, it may be limited by false positive results, and invasive biopsy is often needed to confirm suspicious cases.
In order to evaluate the value of AI model designed to assist these cases, the researchers collected data of 491 patients with non-small cell lung cancer, who were imaged before treatment with analog PET/CT scanner or digital PET scanner. According to the scanner used, patients were divided into training queue and verification queue. There were 385 people in the simulation training and testing queue and 106 people in the digital verification queue.
Forty clinical variables, tumor features and image variables, such as F-18 FDG radioactive tracer uptake (SUVmax) of lymph nodes, were collected, and different combinations of variables in several machine learning methods were compared. The researchers chose a gradient enhancement classifier with 10 eigenvalues as the final model.
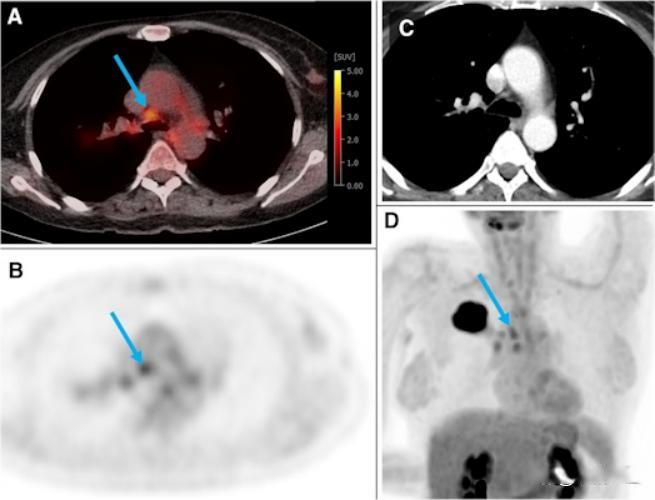
The above picture comes from a 54-year-old woman in a training and testing cohort, suffering from G2 adenocarcinoma in the right upper lobe (44 mm). The uptake of F-18 FDG in several hilar and mediastinal lymph nodes was higher than that in normal liver (PET score was 3). The blue arrow shows N2 paratracheal lymph nodes with a short axis of 7 mm and an SUVmax of 3.5. According to the visual assessment, this case is suspected of N2 disease. According to the gradient enhanced classifier model, the probability of N2/3 is only 0.17. Ultrasound-guided bronchial puncture biopsy of LNs at the same hilum, paratrachea and carina confirmed that the patient was NO. Image provided by European Journal of Nuclear Medicine and Molecular Imaging through CC BY 4.0.
The gradient-enhanced classifier model shows that the AUC in the training and testing queue is significantly higher than the visual PET score (0.91 vs.0.87, p=0.003), and the AUC in the verification queue is slightly higher (0.94 vs.0.91, p=0.023).
It is worth noting that the gradient-enhanced classifier model is superior to the visual evaluation of radioactive tracer uptake in lymph nodes above mediastinum, which is the most common and effective diagnostic threshold in visual F-18 FDG-PET/CT evaluation. They also reported that the coordination of PET images between the two scanners does affect the visual evaluation of SUVmax and lymph nodes, but it does not reduce the AUC of the model.
In order to facilitate further verification, the team has published all variables and machine learning codes as open data and used a web application on GitHub. The network application requires users to input 10 eigenvalues to calculate the prediction probability of N2/3 disease.
Dr. Rogasch and his colleagues said that the research results show a good prospect and deserve further study.
They concluded: "We look forward to the external verification and validity proof in the intervention test."
关于作者